This is the third and final part of our series on OpenRefine, The Outlier team’s favourite tool for cleaning large datasets. OpenRefine is a game-changer when it comes to managing messy data, especially for larger datasets where spreadsheet programs often struggle to keep up.
Here’s what Laura Grant, our managing editor, has to say about it:
It took Alastair two years to convince me to use OpenRefine… My conversion happened when I had a CSV with more than 26,000 school names that was full of typos. The facets and clustering that I dismissed as too complicated saved me hours of time. Since then, I’ve tackled lists of government invoices where information was manually entered by multiple people who added their own twist to spellings of company names and products bought. Now, I can’t understand why I resisted using it for so long.
If you’re still on the fence about using OpenRefine or feel intimidated by its features, this tutorial will walk you through two of its most powerful tools: clustering and editing. These tools will help you clean and standardise your dataset, saving hours of work. Once you give it a go, we can practically guarantee that you won’t look back.
Watch the video
Let’s get started!
Correcting data using text facets
A facet works a lot like a filter. Faceted browsing allows you to explore and filter data dynamically. Clicking on the dropdown arrow in the column header, select Facet > Text facet. This will display all the unique values in the column – and will help you identify errors.
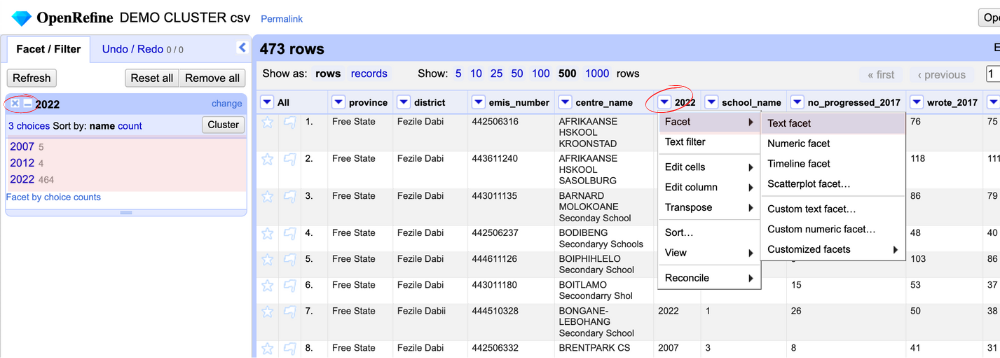
For instance, we can see that someone has mistakenly input 2017 instead of 2022. Click on the 2017 in the facet box in the left panel. This will isolate the row(s). Hover over the incorrect cell. Click Edit, change it to 2022, and click Apply.
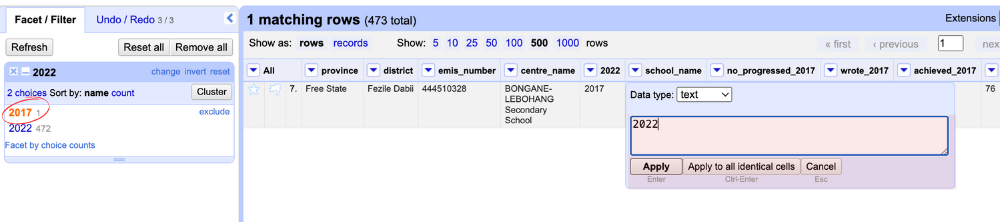
To go back to the full dataset, remove the filtering or facet by clicking on the x on the facet box in the left panel.
Using text filters to edit specific entries
Text filters are helpful when you know there’s a typo – a misspelling or formatting error – you want to correct.
To apply a text filter, select the column where the typo might exist. Click on the dropdown arrow and choose Text filter.
Now find and fix typos. In our example, the province name ‘Free State’ has been entered as ‘free state’ in some instances. Type the correct formatting or spelling into the text filter, and press Apply.
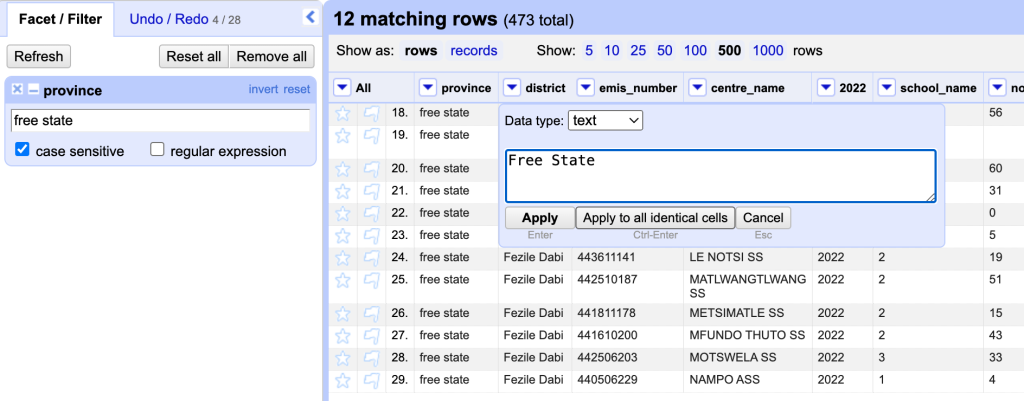
- To edit one occurrence: Hovering over the cell. Click Edit, change it to ‘Free State’, and click Apply.
- To edit all matching cells at once: Use the option to Apply to all identical cells and make batch corrections.
Close the filtering by clicking the x in the lefthand panel.
Replacing values in bulk
It’s pretty straightforward to standardise inconsistencies such as mixed abbreviations. In our example, we want to replace ‘SS’ in the centre names to ‘Secondary School’.
Here’s how:
Click the column’s dropdown arrow. Select Edit cells > Transform > Replace.
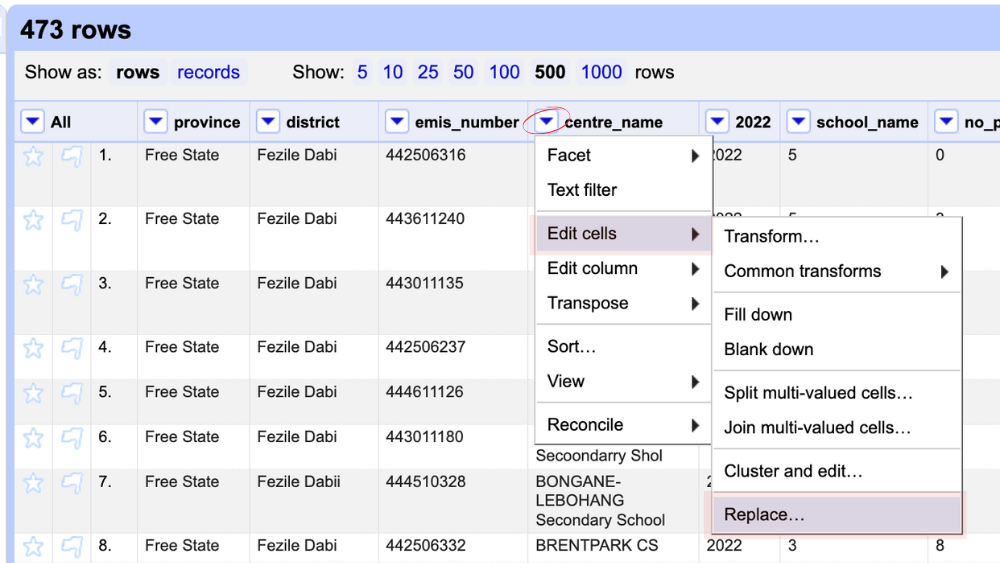
To replace all occurances of ‘SS’ with ‘Secondary School’, enter ‘SS’ as the value to find and ‘Secondary School’ as the replacement text. I’m using all caps here as the dataset uses caps.
Check the boxes for whole word and make sure case insensitive is NOT checked. Click OK to apply the changes across all rows.
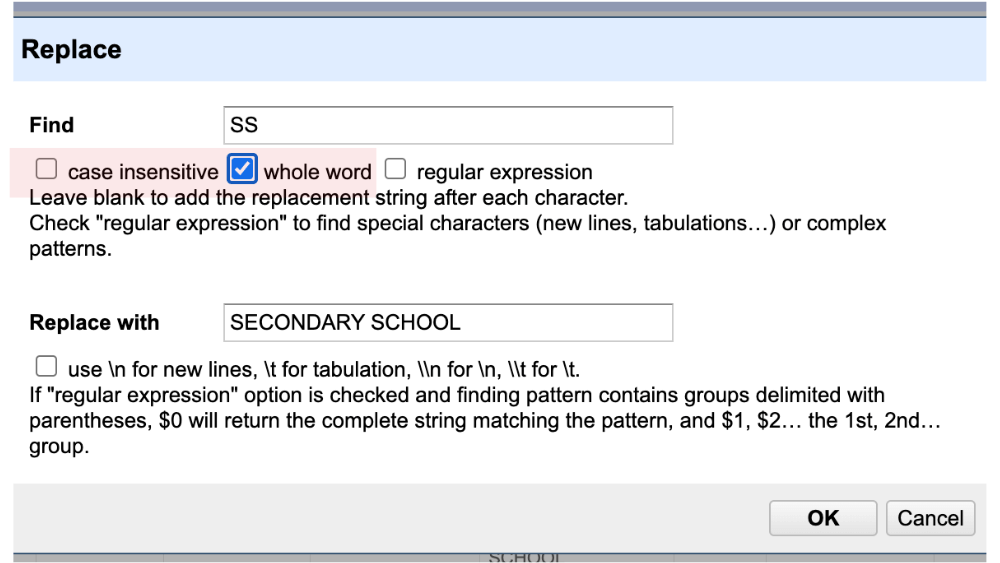
Clustering to detect and fix similar entries
What is clustering? Inconsistencies in your data – such as variations in spelling, typos or different formats for the same information – can wreak havoc on your analysis. But mastering the clustering feature will keep you on the straight and narrow.
Clustering is a tool that identifies variations in similar data entries, allowing you to group and merge them into a single, consistent format. It’s particularly useful for:
- Correcting typos and spelling variations. Quickly spot and standardise inconsistencies.
- Cleaning up data entered manually. Minimise errors from human input.
- Saving time. Automate tasks that would otherwise require hours of manual editing.
In our example dataset that includes province names, there are entries like ‘Free State’, ‘Free States’, ‘free state’ and even ‘FSS’. Clustering can detect these as related entries and help you standardise them in just a few clicks.
How to use clustering
First, cluster your data: Click the dropdown arrow in the relevant column (eg, province). Select Edit cells > Cluster and edit.
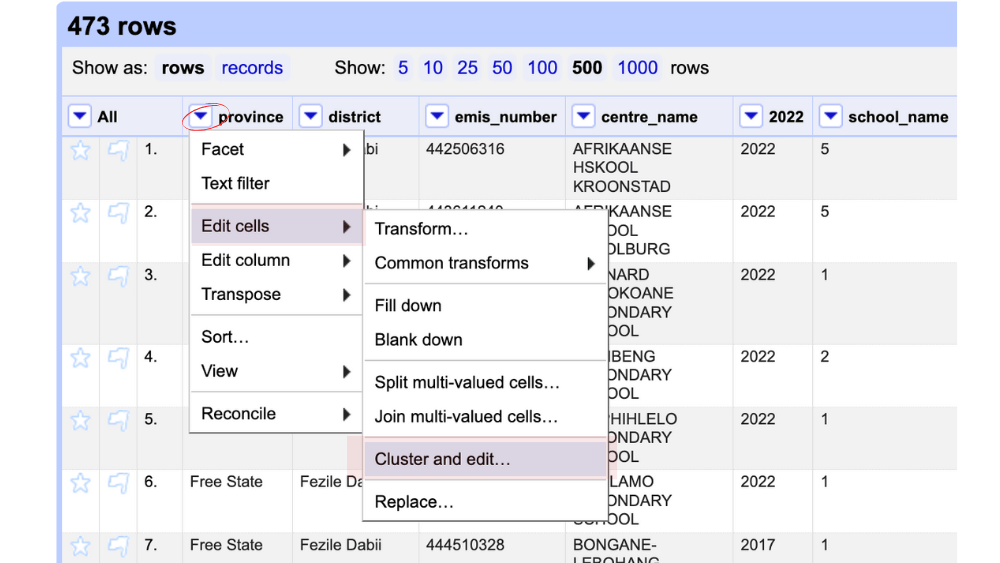
Choose a clustering method: In the clustering dialogue, you’ll see options for:
– Key collision. These are groups that are likely to have been typed differently but are intended to be the same – eg FS vs FSS.
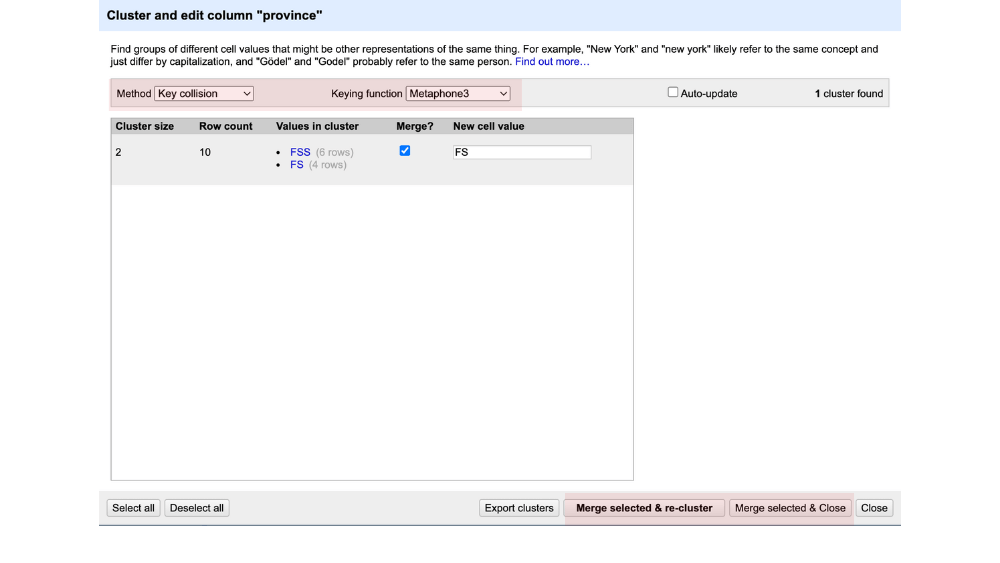
– Nearest neighbor. This focuses on similar-sounding or visually similar entries – eg Free State vs Free States.
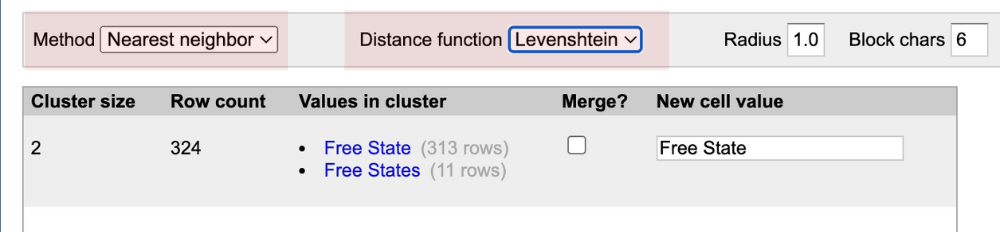
For most datasets, the Key collision method with the Metaphone 3 keying function works best. It’s considered the most advanced option for identifying related entries.
Now review suggestions, such as FS vs FSS. Select the correct value (eg, FS) and click Merge selected and close.
- Use Merge selected and close when you are sure that the clusters currently displayed are sufficient for your cleaning task.
- Use Merge selected and re-cluster if the dataset has many related or cascading inconsistencies that might not all appear in the initial clustering.
Verify the changes by using a text filter to confirm that the incorrect value no longer exists – eg filter for ‘FSS’ to check that all instances have been replaced with ‘FS’.
Exporting your cleaned dataset
Once you’ve finished editing, export your dataset. Click Export in the top-right corner. Select your preferred format, such as CSV. The file will download automatically and can typically be found in your downloads folder.
The full series
Part 1: Installing OpenRefine and merging datasets | WATCH
Part 2: Removing duplicates and tracking changes | WATCH
Part 3: Fixing inconsistencies with the cluster and edit tool | WATCH
Notebook
- OpenRefine’s official website and documentation
- Read more: 5 reasons to switch to OpenRefine to clean data
- Subscribe to The Outlier’s YouTube channel to be notified of new updates
- Sign up to The Outlier’s newsletter for weekly tools, tips and data-driven insights